The Impact of ARIMA Models on Architectural Business Forecasting
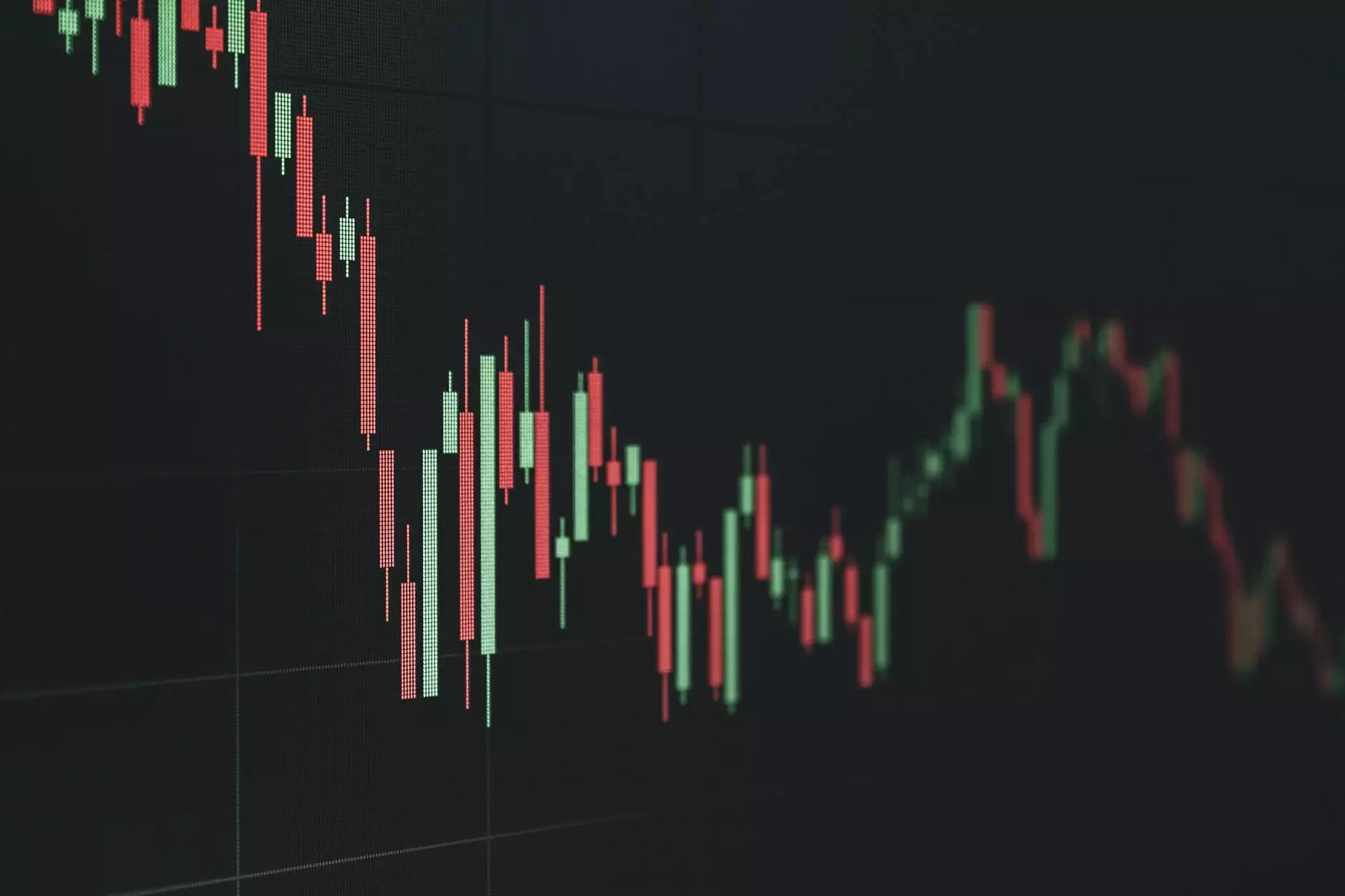
In the fast-paced world of architecture, making informed decisions is crucial for staying ahead of the competition. One of the powerful analytical tools used in this domain is the ARIMA model, which stands for AutoRegressive Integrated Moving Average. This statistical technique is pivotal for time series forecasting, allowing architects to predict future trends based on historical data.
What is an ARIMA Model?
The ARIMA model is a sophisticated yet accessible statistical method used predominantly in time series analysis. Its primary function is to understand and predict future points in a series data based on its own historical values. The model is a combination of three main components:
- AutoRegressive (AR): This component indicates that the current value of a series can be explained by its previous values.
- Integrated (I): This part refers to differencing the raw observations to make the time series stationary.
- Moving Average (MA): This element involves using past forecast errors in a regression model to predict future values.
Why Use ARIMA Models in Architectural Forecasting?
Architects face numerous challenges, including market fluctuations, changing consumer preferences, and resource allocation. Implementing ARIMA models can provide key benefits, including:
- Accurate Forecasting: By analyzing historical project data, ARIMA models help architects predict the future demand for their services efficiently.
- Strategic Planning: ARIMA aids in long-term planning and decision-making by revealing trends and potential market shifts.
- Resource Management: Understanding future needs can help firms allocate resources more effectively, minimizing waste and optimizing budget expenditures.
The Architectural Application of ARIMA Models
Implementing ARIMA models in the architectural field can lead to significant advantages. Below are some specific applications:
1. Project Demand Forecasting
Architectural firms can use ARIMA models to forecast demand based on historical data, such as previous project sizes, types, and client demographics. By understanding these patterns, firms can tailor their marketing and service offerings to better align with anticipated needs.
2. Budget Forecasting
Every architectural project has its budget limitations. Analyzing past expenditures with ARIMA models allows firms to predict future costs more accurately. This foresight provides a framework for more effective budgeting and financial planning.
3. Resource Allocation
With accurate forecasts, architectural firms can optimize employee workload and allocation of materials, reducing downtime and improving overall efficiency.
Steps to Implement ARIMA Models
To successfully implement ARIMA models, architects should follow these key steps:
Step 1: Data Collection
The first step involves gathering historical data relevant to the firm’s previous projects. This data can include project timelines, costs, resource use, and client feedback.
Step 2: Data Preprocessing
Ensure the data is clean and formatted correctly. This might include handling missing values, removing outliers, and possibly transforming variables to fit the assumptions required by ARIMA.
Step 3: Model Selection
Determine the appropriate model parameters (p, d, q) through techniques such as the Autocorrelation Function (ACF) and Partial Autocorrelation Function (PACF) plots.
Step 4: Model Fitting
Fit the chosen ARIMA model to your historical data using statistical software packages that support time series analysis.
Step 5: Model Validation
Evaluate the model’s forecasting accuracy with metrics such as Mean Absolute Error (MAE) or Root Mean Square Error (RMSE). Validate using a hold-out test set.
Step 6: Forecasting
Once validated, leverage the model for forecasting future projects, expenses, and needs, continuously updating the model with new data as it becomes available to improve accuracy.
The Future of ARIMA in Architectural Practice
As the architectural industry becomes increasingly competitive, the integration of advanced forecasting models like ARIMA will likely become standard practice. By continually refining these models with new data and improving methodologies, firms will be positioned to navigate market uncertainties more effectively, positioning them to capture more opportunities in their field.
Conclusion
In conclusion, embracing ARIMA models in the architectural business landscape can lead to better forecasting and ultimately drive success. By understanding historical trends and making informed predictions, architects can enhance their operational efficiency, improve client satisfaction, and achieve higher profitability. As data analytics evolve, the integration of tools like ARIMA will be essential for architects looking to thrive in a dynamic market.
For more insights on utilizing data-driven forecasting in your architectural practice, explore more at architectural-model.com.
arimodel